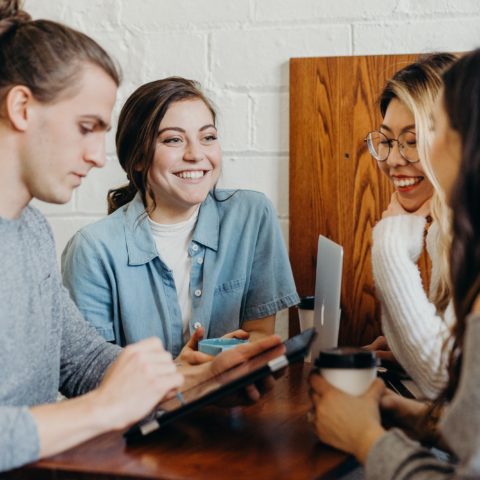
If there were cardinal sins in marketing, I think many would be data related. Think about digital customer experiences that explicitly show that your personally identifiable information (PII) is clearly not put to good use. For me, the one where my middle-names (which I never use) has been concatenated to my first name (without space or hyphen of course) never fails to irritate me every time I receive a marketing communication from that top tier airline which I won’t name …
The fact is, when data is kept organised, tidy and updated regularly it increases productivity and reduces the risk of costly errors. More so, from a CRM standpoint, customers respond much more positively when the information you know about them is correct – which is why data cleansing is so crucial.
Quite simply, it’s not that easy. Even though data management technology is constantly evolving, there is a lot that is common-sense to a human being which is not straight forward to industrialise. Put it another way, the devil is in the detail and there is no shortcut to doing it properly. Added to this are the fact that data sources tend to multiply, that new data keeps being created (on existing and new sources) and that clean data has an expiry date. Many things are conspiring to making that problem a recurring one, but all is not lost provided you have access to the expertise and the tools required for the job! Let’s have a look at our most requested data cleansing practices.
This has become a common challenge because of the multiplication of channels and technical point solutions. Not only does Campaign Management have a database, but so does the Website, the Mobile App, the eCommerce, the POS, the Ad Management system, the optimisation widget, etc… In other words, there are many sources that hold data that could be useful from a marketing perspective whether for insight or CRM execution. The issue here is that this data might not be accessible or actionable where it is. Typically, this calls for consolidating sources into a Marketing Datamart like Adobe Campaign’s database. A Data Cleansing process likely involves finding a way to match-up records from various sources, which can mean complex matching rules when a unique identifier is not available.
Once data is consolidated or moved within a single source, the next challenge might be one of duplicates – i.e. several occurrences of the same consumer. This could occur for a number of reasons. For example, they changed their email address, or have misspelt their name on the mobile App, or perhaps they’ve used their maiden name in a survey, etc… The list is endless, and attention needs to be paid to catching these duplicates at the source during the data creation process – but this may not always be possible. It’s also worth noting that identifying such duplicates is often only half the battle as deciding which data to keep and which to discard during the merging process can also prove tricky.
In the context of data cleansing, normalisation usually refers to making a dataset more coherent, as opposed to the mathematical normalisation of data which can be necessary when looking at modelling or Machine Learning, for example.
To focus on a practical use-case, let’s consider address normalisation. The same postal address could be written on a single line separated by commas or it could be split into several fields such as Line1 and Postcode, however, it could also contain abbreviations like Rd for Road or St for Street. This is why most websites now use real-time validation within the form that the consumer fills in when they register. Unfortunately, the reality of data life means this is not always the case and those issues need to be addressed (if you don’t mind the pun)…
GDPR has been at the forefront of data challenges for the past few years so it won’t come as a surprise that opted-out consumers should definitely not receive marketing communications. That being said, this might not be easy to implement for large companies with multiple brands, multiple levels of opt-in and multiple territories. Aside from the subscription use-case, suppressions might also come in the form of a “gone away” file or sadly a list of people who have passed. There could be many reasons to suppress data, some legal, some business driven or even cost related. Whichever it is, the process of matching data with the correct customers and identifying files remains an important consideration.
In data cleansing, transposition can relate to the transformation or manipulation of data prior to loading into a system like Adobe Campaign or during the creation of a Single Customer View and can take many forms. An example would be to transform multiple rows of xml data into individual flag fields or a single field with a list of searchable values loaded against an individual or record. An example of transposition can be carried out in Adobe Campaign with a Data Management tool called change dimension which lets users change the target dimension and define deduplication criteria. A common use-case for this might be householding. When planning a Direct Mail campaign, the cost implications of poor-quality addresses are quite obvious but even with clean data, it might not make sense to send several letters to the same household. One solution is to look at households instead of people and select the most appropriate contact within each one, according to their title or even Lifetime Value (LTV). Be aware, however, that this conceptually simple solution can sometimes be challenging to implement in practice.
Standardisation typically refers to ensuring format is consistent across and within datasets. Dates are commonly a source of data issues because of formatting inconsistencies. Think about US versus UK format, whether it is just a date or a date and time, or even a full timestamp with several decimals of precision. At best data can be lost, at worst you might advise a client that their appointment is at 14:30:00.000 on the 20-11-12 which is bound to create some confusion… Leaving aside the tricky topic of dates, it is common to have data flags representing a value such as 1 for Mr and 2 for Mrs but another source might hold a “Mr” or “Mrs” as text whilst a third source might hold the full “Mister” and “Missus” (you’d be amazed). When it comes to using data for CRM purposes, the customer experience could be at risk if standardisation has not taken place beforehand.
Finally, our last topic is enrichment which consists of enhancing a marketing dataset with 3rd party sources or with information derived from linked tables. A consumer dataset can, for example, be enhanced with geolocation data from the PAF (Postal Address File) and this, in turn, can enable use-cases around identifying closest point of sale. That same work could also be done through calling the API of a data service provider which is a very different type of solution technically speaking but might provide more precise information such as the driving distance. On the other hand, derived information can be used to make a deep and complex dataset easier to access for the CRM team. For example, a simple RFM score can be derived from the transactions table. There is no shortage of use-cases when it comes to creating additional scores and attributes.
If this overview of a few data challenges and associated data cleansing practices resonates, it is time to clean!
Even if you feel these challenges don’t apply just yet, don’t forget that as a rule of thumb, 20% of CRM data expires on a yearly basis… And if you have not looked into data quality recently, the above might give you some ideas about where to start.
Whichever way, if you’d like to discuss your requirements please get in touch.