Insight
The Role of AI in CXM
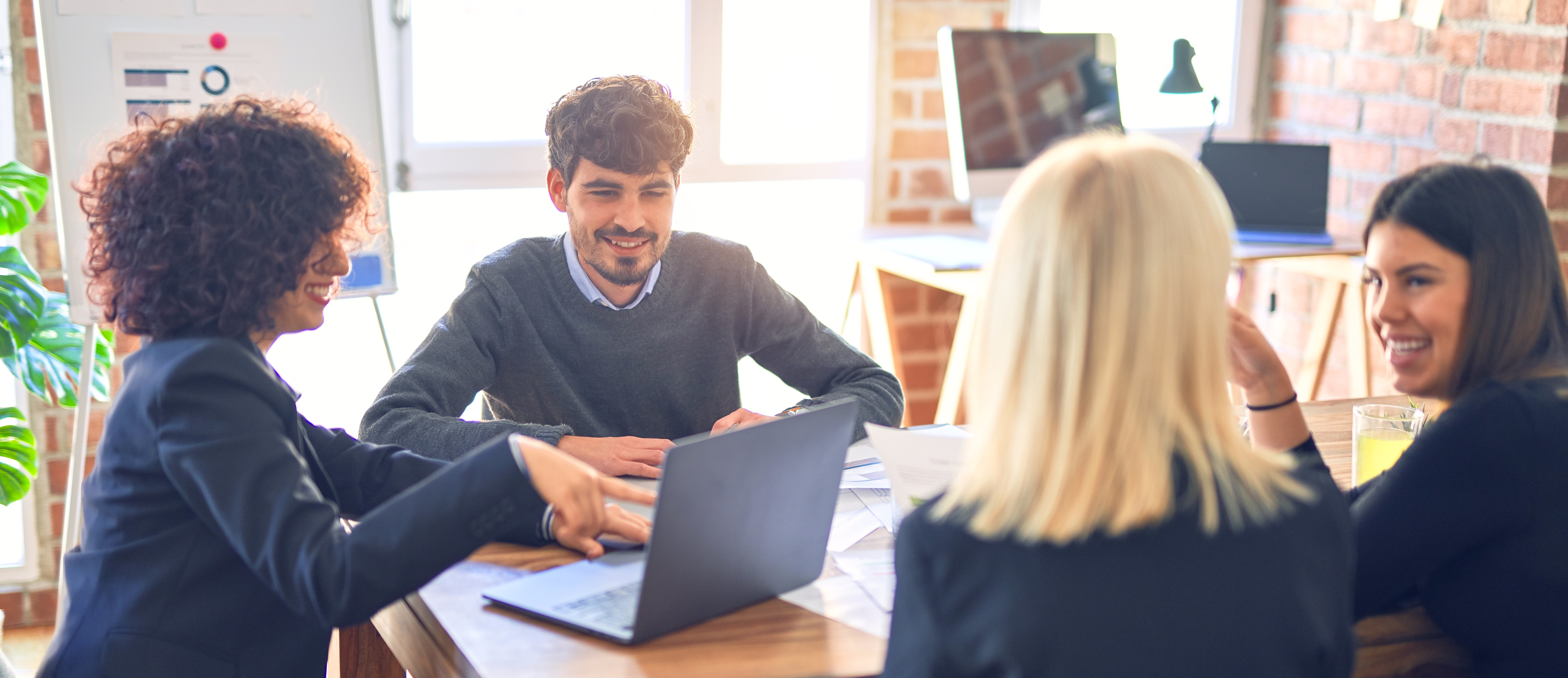
21 January 2025 Marketing Technology
Yes, it’s another blog about AI. Not the AI you’re familiar with, but one that will change everything you think you know about martech. We’re less interested in AI-generated creative and more interested in the future of AI for analytics, data processing, decisioning and personalised marketing at scale.
Table of contents
Another blog about AI? Really?
We know. But this one is different. We’re less interested in AI-generated creative and more interested in the future of AI for analytics, data processing, decisioning and personalised marketing at scale.
If that sounds less exciting than chatbots and image generators, it’s not. This is AI with real potential to give your business a competitive edge. Making the right moves now – building capabilities, establishing processes and safeguards, and investing in AI marketing tools – could be the difference between success and stagnation. So, yes, it’s another blog about AI. Not the AI you’re familiar with, but one that will change everything you think you know about martech.
The role of AI in marketing and CXM
Artificial Intelligence (AI) technologies emulate human processes and abilities. It’s the same core idea whether we’re talking about generating text, surfacing insights from data sets, or automating work. Large data sets + iterative processing algorithms + advanced pattern identification = outputs that mimic human ability. Add the capacity to learn from feedback, and you’ve got a piece of tech that seems able to make decisions for itself. There are two places that AI tools turn up in CXM (customer experience management):
Background processes: Machine learning
For years, “AI in marketing” has been synonymous with data models used behind the scenes. These machine learning (ML) models could analyse vast amounts of customer data, identify patterns, and make predictions about future behaviour. They enabled marketers to improve efficiency and personalisation without deep technical expertise.
-
- Predictive analytics: Using big data models to segment databases and forecast customer behaviours and preferences based on historical patterns and variables.
- Recommendation engines (next-best-action): Identifying the most relevant offer, message or service to deliver based on the customer’s propensity to act and the value it could generate.
- Propensity and risk models: Gauging a customer’s likelihood to respond positively to experiences, products or offers, and the associated risks.
- Adaptive modelling: Continuously updating ML models based on real-time customer responses to refine recommendations and insights.
- Product recommendations: Suggesting suitable products or services based on behavioural patterns and purchasing affinities.
While these capabilities were a massive leap forward in marketing, they operated largely in the background. Marketers often relied on data teams to build, deploy and interpret data models.
Foreground creative tasks: The rise of GenAI
By leveraging large foundational models trained on vast datasets, generative AI (GenAI) can churn out content, ideas and strategies. With large language models (LLMs) like OpenAI’s ChatGPT and Google’s Gemini, and generative tools like Adobe Firefly and Midjourney, marketers no longer need coding expertise to interact with AI or harness its potential.
-
- Creativity at scale: From marketing content to product ideas, GenAI empowers teams to explore creative possibilities faster and more comprehensively.
- Personalisation: GenAI can use insights from background algorithms to create highly customised customer experiences, messages, and offers.
- Efficiency and cost reduction: Automating tasks like content drafts, emails or administrative summaries saves time and allows marketers to focus on more valuable work.
- Automated customer service: AI-driven chatbots generate instant, tailored responses to customer inquiries – although the results have been a mixed bag so far.
- Innovation and competitive advantage: Businesses can leverage GenAI to bring campaigns to market faster than ever.
As GenAI improves, it’s likely to automate more tasks and lessen marketers’ admin workloads. Natural language chatbots like GPTs (Generative Pre-trained Transformers) also mean that you don’t need coding knowledge to manipulate models or interact with data.
Still, there’s a reason that GenAI gets mixed reviews from marketing pros. Skip ahead to learn about the risks of AI in marketing.
How AI helps marketers solve 6 common business challenges
1. Create a single customer view
AI can stitch together fragmented data to de-anonymise visitors. This helps you understand customer behaviour and build comprehensive profiles. Although some de-anonymisation tools might cause GDPR headaches, others focus on customer value.
-
- Zeta Marketing Platform uses 12.7B digital identifiers and 2.5k+ real-time signals to identify high-value customers and optimise their experience in real time.
- Adobe Real-Time CDP (Customer Data Platform) leverages AI to normalise known and pseudonymous customer data collected from online and offline touchpoints.
- Apteco FastStats uses AI to segment audiences, find lookalikes and predict buying behaviour. Our extensive experience with FastStats has helped our clients connect multiple data sources to gain actionable customer insights.
As well as creating holistic and progressive profiles, these capabilities help you maintain a clean database.
2. Personalise marketing for better results
Customer decisioning AI data models analyse large volumes of data to detect patterns. These patterns can predict which channel, content, product, or message will resonate most with each customer.
This is incredibly valuable for creating personalised experiences at scale and in real time. AI tools can then dynamically tailor email content, website experiences and even app interfaces for each user.
Most marketing automation platforms (at least the good ones) feature these capabilities to some extent. They tend to veer slightly in one direction, so it’s important to clarify your use case first. For example:
-
- BrazeAI™ is popular among B2C brands with a strong mobile presence
- Bloomreach leverages AI to enable e-commerce brands to personalise shopping experiences
- Adobe Target uses AI for A/B testing, personalisation and automation at the enterprise scale
- HubSpot offers AI features across content, sales, customer agent and social media functions
3. Make ad budgets work harder
AI is foundational to Google Ads. For many years, it has been quietly helping in the background, supporting advertisers in maximising their time and return on investment.
Machine learning has long been pivotal in bidding for and serving online advertisements. AI evaluates campaign performance in real-time, ensuring every dollar delivers maximum impact. Virtually every platform uses this ‘programmatic’ advertising model.
But the one to watch is Bing. Many of the emerging GenAI tools (Copilots, Gemini, Arc Search and others) are currently tied to Bing, Microsoft’s long-suffering Google search competitor. Microsoft Advertising is already rolling out features like conversational ads and chatbot APIs that could help you engage audiences in a different way.
4. Track and measure cross-channel customer journeys
Advanced analytics enables marketers to track and measure complex customer journeys with new granularity. AI-powered reporting and attribution modelling help to surface insights and guide decision-making. Essentially, AI marketing tools shortcut data analysis workflows to:
-
- Map touchpoints from initial awareness to final conversion
- Surface actionable insights faster from large data sets
- Measure the impact of marketing efforts on conversions, behaviour and revenue
- Identify bottlenecks and optimise channel performance
- Measure the value of multi-channel campaigns more accurately
Adobe’s Attribution AI feature is a great example of the difference between regular data processing and AI. Attribution AI quantifies the impact of passive and active touchpoints, so you can optimise campaigns to generate the most value. For smaller and growing brands, Tableau is an industry-leading BI and data visualisation tool that’s often more cost-effective than Adobe counterparts.
5. Diagnosing issues and anomalies
AI can identify unusual patterns in marketing data, such as sudden drops in traffic or conversion rates. In fairness, you can probably do that, too, by looking at reports. Where AI helps is by pinpointing the underlying causes of issues and anomalies so you can take corrective action. Adobe’s Contribution Analysis is a good example. Available in Adobe Analytics, Contribution Analysis is a machine learning process designed to map the contributions to observed anomalies. Most web analytics platforms offer some version of error or anomaly detection. But without the data-processing capabilities of ML algorithms, diagnosing and fixing the issue is often a matter of trial and error.
6. Accelerate business processes
AI-powered automation is improving speed and accuracy in asset handling, approvals and task management. Most major vendors are rolling out generative Copilot tools to help you speed up daily tasks. But back-end automations are just as important for efficient work. One solution currently in the spotlight is Adobe Workfront. Taking inspiration from resource optimisation and project management software, Workfront brings automation and resource management to marketing. It’s not the first of its kind, but it’s an Adobe solution, so you know it’s got oomph.
The limitations of AI in marketing
AI tools are definitely impressive. And they undoubtedly surpass human capabilities in terms of speed, scale and volume. But are they actually ‘intelligent’? Most experts would say no.
Don’t worry – we’re not digressing into philosophy. The point is that all AI tools are designed and trained for a specific task. ChatGPT might seem capable of adaptive thinking, but it’s trained to provide confident, human-like answers.
Similarly, Adobe Customer Journey Analytics can predict your customers’ next move faster and more accurately than you could ever hope to – on a scale beyond human comprehension. But it’s not trained on website content. It can lead them to your landing page, but it can’t write the content.
The computer scientist and co-creator of VR technology, Jaron Lanier, summed it up for The New Yorker:
“The most pragmatic position is to think of A.I. as a tool, not a creature. It’s easy to attribute intelligence to the new systems; they have a flexibility and unpredictability that we don’t usually associate with computer technology. But this flexibility arises from simple mathematics.”
Getting started with Generative AI
We see three levels of adoption for GenAI:
-
1. Off-the-shelf tools ($)
Use an existing foundational model(like ChatGPT) directly by inputting prompts directly into its interface.
Depending on the use case, you can speed up tasks like drafting emails and subject lines, brainstorming ideas, writing job descriptions or conducting broad research.
-
2. Prompt engineering ($$)
Leverage existing models by programming your own applications and connecting them via API, providing additional context (data, templates or example outputs) to the output you want to achieve.
This allows you to use public large language models (LLMs) while protecting your IP and generating more useful outputs.
-
3. Customising models ($$$)
Fine-tune existing foundational models by adding layers of proprietary data to significantly alter how the model works. Given the enormous amounts of data in foundational models, any data you introduce needs significant scale. This makes customisation expensive and potentially prohibitive without a solid long-term business case.
What’s the catch? The risks of AI in marketing
Accuracy
GenAI still generates incorrect or misleading information. These ‘hallucinations’ can seem plausible until you fact-check the content. Machine learning algorithms, by design, are less prone to hallucinations. However, they can still yield insights that don’t quite fit your use case or misinterpret nuanced data.
Data privacy and security
AI relies on vast amounts of data, including sensitive customer information. Data breaches and leaks can have severe consequences for businesses. There are also ethical questions around how AI collects and analyses data. Privacy, consent and transparency are increasingly important for users.
Bias
AI algorithms can perpetuate biases present in the data they’re trained on. They may inadvertently reinforce stereotypes and prejudices, particularly in areas like advertising and content generation.
Skills gap
The rapid evolution of AI requires marketers to continually upskill and adapt to new technologies, or risk becoming obsolete. We’re not so concerned about the job losses that some pundits predict. There will always be plenty of work for switched-on marketers. Automating low-level and repetitive tasks is more like an opportunity to upskill or retrain.
Dependency
Excessive reliance on AI can stifle creativity and innovation. AI-generated content also tends to lack the emotional connection and authenticity that human-created content can provide. This is why it’s so important to remember that AI is a tool, not a colleague.
Evolving regulation
The EU AI Act, introduced in 2023, is likely the first of many laws that will restrict or reshape AI in marketing. For example, the Act says: “Content that is either generated or modified with the help of AI…need to be clearly labelled as AI generated so that users are aware when they come across such content.”
You must make an effort to stay informed about changing regulations around AI use.
To mitigate these risks, it is essential to:
-
- Set limits for responsible AI use within your teams
- Educate your organisation on the uses and limitations of AI tools
- Use high-quality, unbiased data to train AI models
- Implement robust data privacy and security measures
- Monitor AI systems for bias and take corrective action
- Foster a culture of ethical AI use
- Continuously upskill and reskill employees to adapt to the evolving AI landscape
- Maintain human oversight and control over AI-driven decision-making
The future of AI in marketing and CXM
We expect that AI models will become more accessible for marketers in the coming months. Vendors outside the enterprise sphere are racing to roll out AI features. The next phase will see AI tools mature. As they evolve and the capabilities improve, you can expect to see efficiency gains and cost reductions in daily tasks. Specialised tools and features will follow, enabling AI tech vendors to differentiate themselves in a crowded market.
This is where your business goals become important
AI is going to grow up fast. We recommend revisiting your strategies to see where the evolved versions of today’s AI solutions can add value. Don’t get swept up in the hype when there are bigger opportunities to scale your reach and capabilities using AI tools. This could be your opportunity to get ahead of the competition. While others are playing with GenAI, focus on scaling analytics, collecting first-party data and building a loyal customer base. In other words, invest in customers first. AI marketing tools come later.